How we are using machine learning to detect GOV.UK feedback spam
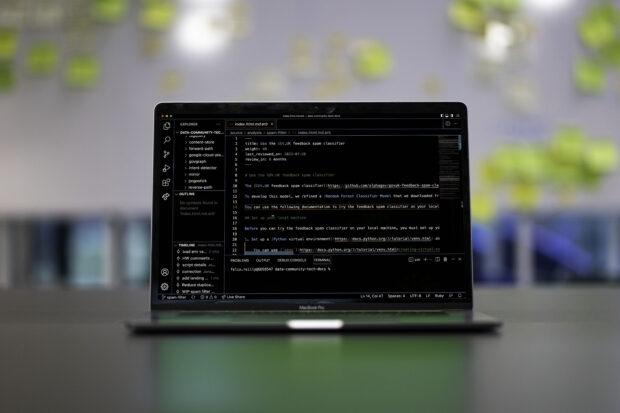
The GOV.UK feedback form was receiving a lot of spam requests. We developed a machine learning model to detect spam responses — here is how we created it.
The GOV.UK feedback form was receiving a lot of spam requests. We developed a machine learning model to detect spam responses — here is how we created it.
The Race Disparity Unit at the Cabinet Office Equalities Hub have analysed different approaches taken by national governments to understanding how they compare on issues such as ethnic diversity and cultural identity.
Richard Laux outlines the 3 main reasons for collecting data about people’s ethnicity and identifies 6 principles for collecting these to meet all users’ needs.
Find out about the role of data specialists in GOV.UK who helped their colleagues to locate accessibility issues across GOV.UK, and how data processing techniques were used to contribute to fixing these problems
The Data Standards Authority team are creating a common data infrastructure across government to improve services for users. Here’s how they’re doing it.
Discover how DSTL are able to share data with partners, by using synthetic data generation techniques to remove or obscure sensitive data while retaining its structure and characteristics
Rachel Beardmore explains how the Race Disparity Unit is making more data available to support research into the effects of coronavirus (COVID-19) on people from ethnic minorities.
We recently held our first completely remote Data Science Community of Interest event. Hillary and James talk in this post about the event and recap some of the presentations
Today the Race Disparity Unit has published the first in a new series of methods and quality reports. Find out more in this blog post
The Race Disparity Unit has recently published the second in a series of summaries of data from the Ethnicity facts and figures website. Discover how the collection of staff ethnicity can be improved